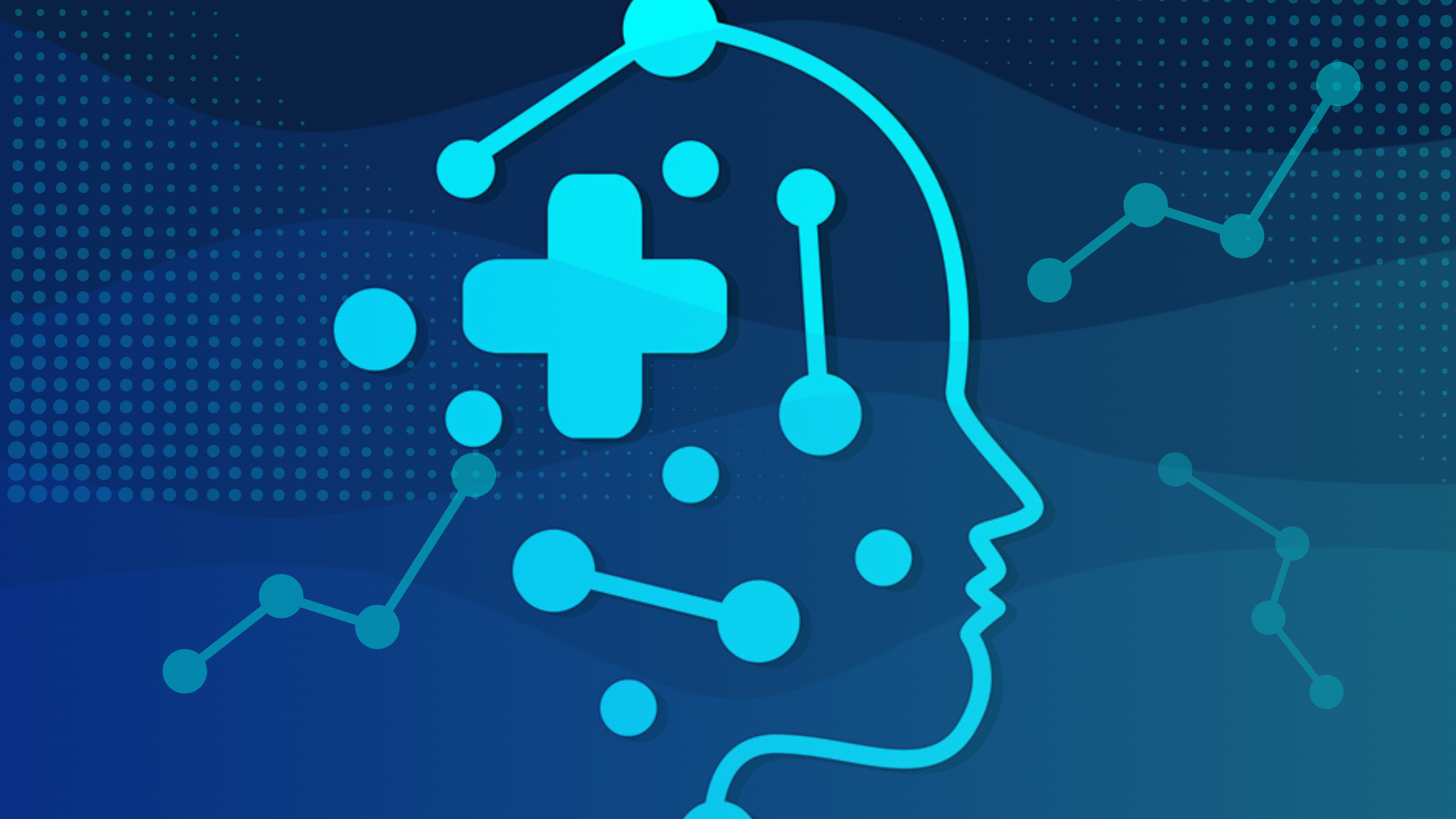
With most of the direct impact of Covid-19 now in the rearview mirror, the worsening mental health crisis in the United States has come into sharp focus. The National Institute of Mental Health (NIMH) reports that one in six U.S. adults live with a mental illness, while Johns Hopkins Medicine adds that 1 in 4 U.S. adults suffer from a diagnosable mental disorder. Data from Mental Health America also reveals a notable increase in the number of people reporting signs of anxiety and depression since the beginning of the pandemic.
These statistics highlight the gravity of the mental health crisis in the U.S. and underscore the urgent need for comprehensive, accessible, and effective behavioral health solutions. But behavioral health plans are confronted with various distinct obstacles in delivering effective care, such as the absence of seamless integration between physical and mental health information and services, the sluggish and inefficient handling of data, and the absence of real-time tools for coordinating care among diverse stakeholders.
Fortunately, the rise of artificial intelligence (AI) and data science-enabled tools in healthcare is paving the way for groundbreaking advances, particularly in behavioral health. By leveraging these innovative technologies, behavioral health plans are now better equipped to identify high-risk members, prioritize their needs, and determine appropriate next steps. In this article, we explore several ways data science and AI enable behavioral health plans to achieve their goals more effectively.
Identify and Prioritize High-Risk Members
By leveraging innovative AI-based approaches, such as machine learning and predictive modeling, behavioral health plans can effectively target individuals who are at a higher risk of developing or exacerbating mental health conditions. This early intervention is vital as it can prevent situations from worsening or becoming critical. High performing systems have proven to significantly increase the number of members identified for care management, leading to improvements in engagement and active cases managed.
AI has the capability to analyze vast amounts of data, including medical records, social determinants of health (SDOH), and other relevant information. By identifying patterns and correlations within this data, AI systems can predict which individuals may be at risk for certain mental health conditions. Moreover, AI can track the correlations between physical and mental health, allowing for intervention and treatment recommendations that are tailored to the individual's specific needs.
Over time, AI systems continuously learn from the data they analyze, improving their predictive capabilities and becoming better at accurately identifying at-risk individuals. This ongoing learning process enhances the effectiveness of early intervention strategies, enabling behavioral health plans to provide timely and targeted support to those who need it the most.
Leverage a Unified Member Profile
To further enhance the delivery of behavioral health services, it is essential to bridge the gap between mental and physical health information and services. Behavioral health plans often struggle with the fragmentation of care, resulting in missed opportunities for early intervention and disjointed treatment. Moreover, in several states across the U.S., mental and physical health are often treated as separate entities, creating further complexities in delivering effective behavioral health care.
However, with the help of a data science-driven platform that aggregates and integrates data feeds from different participating plans, healthcare teams can get a comprehensive and unified perspective of each member. Important metrics like emergency room visits, overdoses, and medication management effectiveness can be easily tracked and securely shared among stakeholders, including healthcare providers, care managers, and payers. This collaborative approach is especially critical as it ensures that all parties involved in the member's care – including home health providers, counselors, food delivery services, and others - have access to the same information to make informed decisions.
In addition, a unified member profile helps to identify gaps in care and prevent unnecessary duplication of services. By having access to complete medical and behavioral health records, healthcare teams can identify missed appointments, lack of follow-up care, or inadequate treatment for comorbid conditions. This proactive approach allows for timely interventions, ensuring that member needs are consistently met.
Develop a Personalized Intervention Strategy
Initiating personalized, holistic behavioral health involves using advanced, data-driven technologies to create individualized care plans for patients. Automation can streamline the process of collecting and analyzing patient data, reducing the time and effort required by healthcare professionals. Different types of data can be gathered from various sources such as electronic health records, SDOH data, and even patient self-reports. Machine learning algorithms can then analyze a member's behavioral history to identify patterns or trends that might not be immediately apparent to human observers. These patterns can help predict future behavior or potential risks, allowing for early intervention.
In addition, data science can be used to analyze therapy outcomes and comorbidities (the presence of two or more diseases in a patient). By examining this data in a comprehensive manner, the care team can gain insights into what treatments have been most effective and how other physical health conditions may be impacting the patient's mental health.
Based on the insights gained from automation, machine learning, and data science, a personalized intervention strategy can be developed. This strategy is tailored to the individual's unique needs and circumstances, making it more likely to be effective.
Analyze Gaps in Care
One of the key advantages of employing data science and AI in behavioral health is the ability to analyze gaps in care with precision and speed – even on a daily basis with the most sophisticated systems. By continuously evaluating the care being provided through real-time data analysis, the system can identify any areas where the patient's needs are not being fully met. For instance, it might spot patterns of missed appointments with a psychiatrist, lack of follow-up care after a significant change in medication, or inadequate or incorrect treatment for comorbid conditions like depression in a patient also dealing with diabetes.
Care plans must also meet the unique and dynamic requirements of the health plan, taking into account a variety of state and/or plan-specific care gap measures that span both behavioral and physical health measures. For example, a plan in California may have very different requirements for mental health follow-ups compared to a plan in New York. Sophisticated algorithms can be used to analyze patient data and track care plan adherence to these requirements.
Machine learning, a subset of AI, can be trained to recognize when a patient's care deviates from the standard set by their specific health plan and make recommendations for next-step actions, allowing healthcare providers to take corrective action swiftly. For example, if a patient consistently misses therapy appointments, the system can generate automated reminders or even schedule alternative modes of treatment, such as telehealth sessions, to ensure that the patient's progress is not hindered.
Finally, predictive analytics, another aspect of data science, can forecast potential future care gaps based on historical data. If the system identifies that patients with certain characteristics are more likely to miss follow-ups, it can preemptively suggest interventions to prevent this.
Determine Next-Best Actions
The wealth of data generated in mental health settings presents an opportunity for AI tools to provide valuable insights that can guide healthcare professionals in making informed decisions about the best course of action for each individual. By analyzing therapy outcomes and comorbidities, AI algorithms can identify which treatments have been most effective for similar patients with similar conditions. This information can guide healthcare professionals in selecting the most appropriate treatment plan for each individual, increasing the likelihood of positive outcomes.
In addition to treatment decisions, AI can also help determine the next-best actions for care coordination and resource allocation. By analyzing the data on gaps in care, AI algorithms can identify areas where additional support or resources may be needed. For example, if there is a high rate of missed therapy appointments in a particular geographic area, an algorithm can suggest strategies to improve appointment attendance, such as implementing reminder systems or offering alternative modes of treatment. This information can be further complemented by data science models that help predict the likelihood that a member is to engage for care management.
With the help of intelligent automation, healthcare professionals can then focus on delivering timely and effective care to high-risk members. The system can identify and flag urgent cases, ensuring that they receive immediate attention. For example, if a member exhibits signs of escalating mental health conditions or is at risk of a crisis, the automation system can prioritize their case and alert the appropriate care team members.
Intelligent automation also assists in coordinating care across different providers and stakeholders involved in the member's treatment. By automatically populating work queues, the system ensures that all relevant parties have access to the latest information and can collaborate seamlessly.
About Vital Data Technology
Vital Data Technology's dynamic, cloud-based Affinitē Solution for Behavioral Health Plans leverages data science and AI-embedded automation to predict outcomes and automate actions in real time, bridging the gap between stakeholders and translating to timely interventions, more meaningful clinical collaboration, and better outcomes for plans, providers, and members alike.
